We know research impact unfolds in complex and unpredictable ways, so how on earth do we learn from and evaluate it? In this blog, I will take a look at some of the approaches we have been developing and using in CECAN - a research centre set up to tackle the issue of complexity in evaluation. I will explain how you can use these approaches to do a quick and effective evaluation of complex research impacts, helping you understand what works and why.
Often, when we talk about evaluation, people’s eyes glaze over with boredom or we become defensive, feeling attacked or unfairly questioned. This is a failure of presentation and perception. What we often fail to recognise, is that evaluation helps us understand whythings have succeeded or failed, it helps us learn. They very same people who get bored or defensive with evaluation, are normally more than happy to have help understanding why something they did worked or not, or learning from past experience, to make today’s endeavours more effective.
Evaluation can deliver this learning, but in most contexts - and indeed in research impact - we don’t have much time or resource for evaluation. It can seem like a luxury or an additional burden. These are important realities; evaluation of research impact should not, and cannot, be overly-long or resource-intensive. It also cannot be something that further fatigues our stakeholders, or bores us as researchers. It needs to be something wholly more appealing, something integrated into our research process, useful in multiple ways, and something which further embeds and enables the long-term empathetic nature of our involvement with research users, stakeholders and publics.
So, some big demands there. Here are a few of the approaches we have been using in CECAN which might enable us to evaluate complex research impacts:
Participatory Systems Mapping: We have been developing and testing this causal mapping technique in a range of settings, from the energy trilemma, to agriculture and the rural economy, to biogas and biomethane production. The approach builds on existing methods (e.g. fuzzy cognitive mapping, theory of change) but with a stronger emphasis on participatory design, and a novel bespoke approach to analysis, using formal network analysis in combination with stakeholders’ subjective views of their system. The maps are networks, made up of factors (i.e. anything which is important in our system, expressed loosely as a variable), and their causal connections. In practice, our systems maps are always built by as diverse a range of stakeholders as possible and designed to capture complexity rather than simplify it away. Building these maps with the stakeholders in your ‘research impact system’, around a mutually interesting topic, can be incredibly useful by itself; they build understanding and consensus, creating valuable buy-in. The maps also serve as a useful planning and evaluation tool too. Particularly for evaluation, we can use them to consider where there are gaps in data or evidence gathering, identify key causal mechanisms we may want to monitor, or use them to inform and refine more focussed theory of change maps or impact planning and tracking tools; they can serve as key (and updateable) resources we return to again and again during and after a research project.
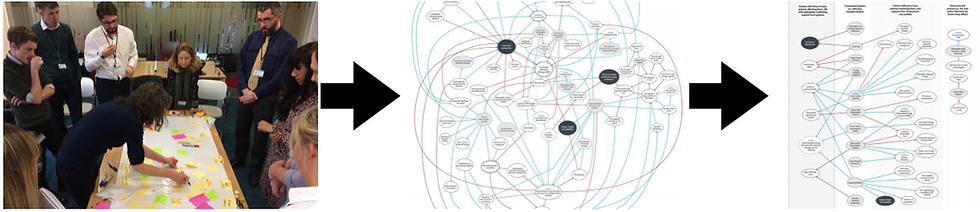
The Participatory Systems Mapping process, from map building, to a full digitised map, to bespoke analysis
Qualitative Comparative Analysis (QCA): QCA is a well-established case-based ‘small-N’ method we have been using with the Environment Agency. It seeks to bridge the gap between qualitative and quantitative data analysis methods and is particularly valuable where complex causation is at play. That is, where combinations (or recipes) of factors lead to important outcomes, rather than all factors having some averaged and standalone ‘net effect’. I won’t attempt to describe the full process here, others have done that very well elsewhere, but key stages include identifying cases (i.e. the things we are evaluating and comparing), defining key attributes and outcomes for these cases, collecting data on these or creating it with stakeholders, and then looking for patterns in outcomes and attributes. The approach is relatively quick and easy, and can be done in a highly-participative manner. This means for research impact evaluation it can be embedded in a research process without becoming a distraction from the research itself, and may even have separate value. Researchers wanting to assess their impact could think about developing the cases, attributes and outcomes for key strands of their research, with their team, or even with stakeholders. Cases might be particular activities or venues for impact which we use, outcomes will likely be perceptions or realities of impact. Here, the output of the analysis will be valuable, but the value of the process of structuring the issue using QCA and exploring assumptions and theories using the analysis, should not be underestimated.
Testing contribution claims with Bayesian Updating: Bayesian Updating is a powerful means of testing claims about impact. It can be a rigorous, disciplined addition to our impact tools and/or complement other approaches aiming to test theories and mechanisms. First, we formulate a claim, i.e. a statement about the contribution research made to an impact or outcome. Next, the method systematically makes use of evidence, logic, prior knowledge, and/or theory, to update our confidence (expressed as a probability) that our claim is true. Researchers are likely to have access to exactly these types of information (evidence, logic, prior knowledge etc), making the approach quick to use. If they don’t have this information, it would be reasonable to assume that collecting and using it would have additional benefits beyond just those of evaluating research impact; the information should be useful in complementing, providing context, and informing other parts of the research. You can read about a high-profile example of this approach being used to assess research impact here.
Those are just three of the approaches we have used in CECAN. Whatever approach we use, the bottom line with complex settings and complex impact is that we need: to be participatory in the way we work, learn and evaluate; to be empathetic in the way we design evaluation and learning activities; and to be in it for the long term, to genuinely realise and learn from our research’s impact, rather than just tick boxes.

Pete is a Research Fellow based at the University of Surrey and hosted by the Centre for the Evaluation of Complexity Across the Nexus and the Centre for Research in Social Simulation. He is currently a UKRI Innovation Fellow working on the appraisal and evaluation of public-private-partnerships (PPP) at the food-energy-water-environment Nexus. Alongside his interest in innovative Nexus PPP, his research interests are in environmental policy, social science, and complexity science. He has used a range of research methods in his research including agent-based modelling of social and policy systems, stakeholder causal mapping, and qualitative and quantitative social research methods. Previously, Pete was one of CECAN's 'Knowledge Integrators', and a Research Fellow at the Policy Studies Institute (PSI). Pete is on Twitter @bapeterj